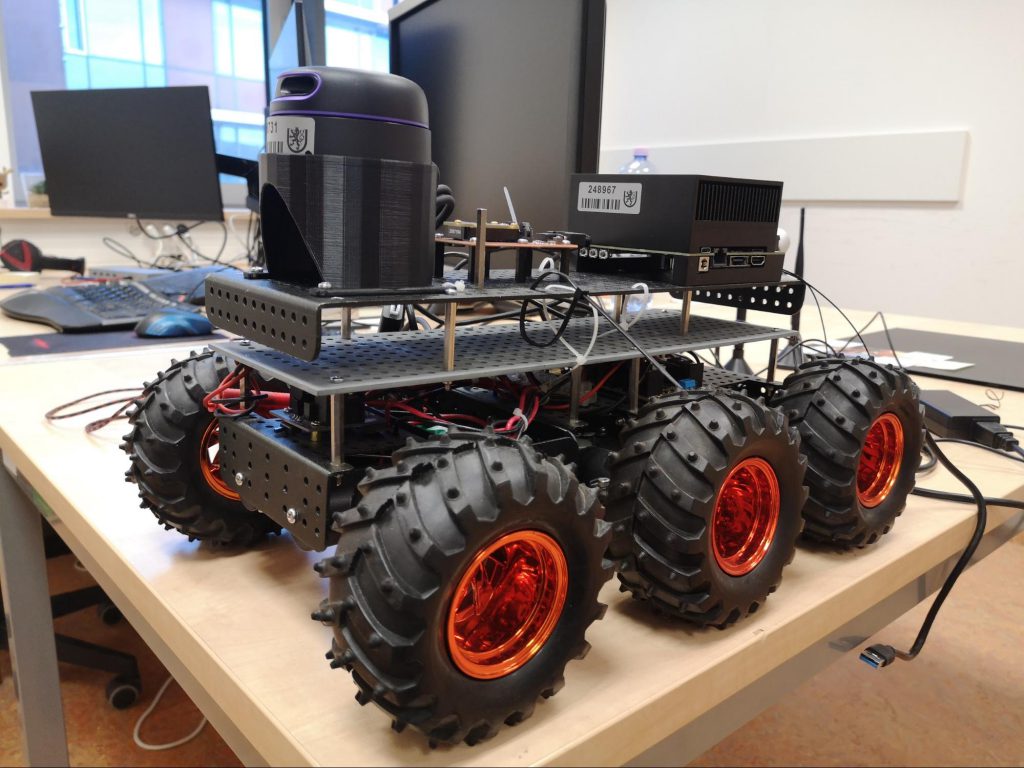
The research of environment classification for mobile robots focuses on using 1D non-visual sensors such as thermometer, humidity sensor, air pressure sensor, or ceiling height sensor to detect the transition between defined environments. The robot’s behavior is then adapted based on this information.
The crucial part of this research is an image classifier trained to recognize the environment’s type. In particular, in our paper [1], we focused on the classification of an indoor and outdoor environment. It is the most extensive comparison of description methods and classifiers (including neural networks) performed on a single dataset to our best knowledge.
In our papers [2] and [3], the system for environment change detection and environment classification is proposed. The system uses non-visual sensors to generate triggers when the transition between environments is detected. Triggers activate the classification of the environment. Thus, its main purpose is to reduce the time requirements of the environment classification compared to the system, which depends only on images.
References:
[1] Neduchal, P., Gruber, I., & Železný, M. (2020, October). Indoor vs. Outdoor Scene Classification for Mobile Robots. In International Conference on Interactive Collaborative Robotics (pp. 243-252). Springer, Cham.
[2] Neduchal, P., & Železný, M. (2020, September). Environment Classification Approach for Mobile Robots. In Proceedings of 15th International Conference on Electromechanics and Robotics” Zavalishin’s Readings” (pp. 421-432). Springer, Singapore.
[3] Neduchal, P., Bureš, L., & Železný, M. (2019). Environment detection system for localization and mapping purposes. IFAC-PapersOnLine, 52(27), 323-328.