Jan Zelinka, Jakub Kanis
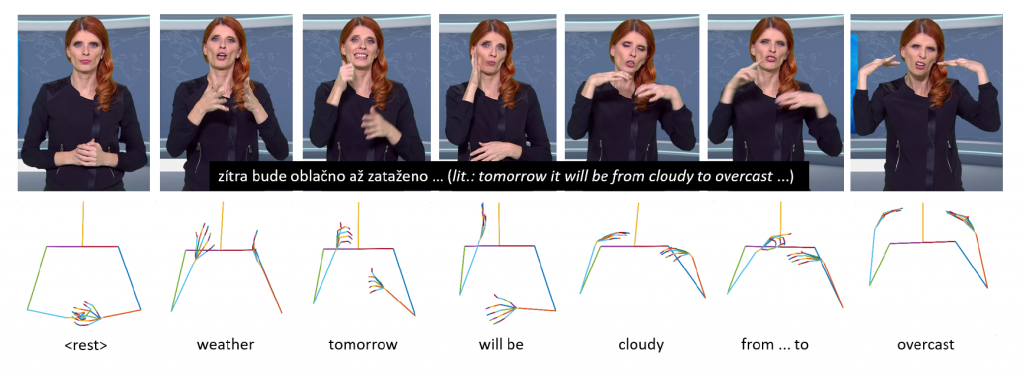
Our work deals with a text-to-video sign language synthesis. Instead of direct video production, we focused on skeletal model production. Our main goal was to design a fully end-to-end automatic sign language synthesis system trained only on available free data (daily TV broadcasting). Thus, we excluded any manual video annotation. Furthermore, our designed approach even does not rely on any video segmentation. A proposed feed-forward transformer and recurrent transformer were investigated. To improve the performance of our sequence-to-sequence transformer, soft non-monotonic attention was employed in our training process. A benefit of character-level features was compared with word-level features. We focused our experiments on a weather forecasting dataset in the Czech Sign Language.
J. Zelinka and J. Kanis, “Neural Sign Language Synthesis: Words Are Our Glosses,” 2020 IEEE Winter Conference on Applications of Computer Vision (WACV), Snowmass Village, CO, USA, 2020, pp. 3384-3392, doi: 10.1109/WACV45572.2020.9093516.